Kendra DeKeyrel is a vice president in IBM’s sustainability software division, specializing in solutions that drive efficiency, reduce environmental impact and create value for organizations around the globe. Opinions are the author’s own.
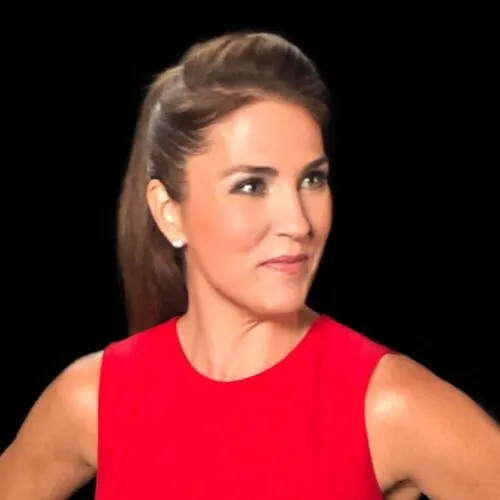
“Sustainability” has different definitions depending on the industry context. In the technology sector, sustainability may refer to building more efficient software. In the financial sector, it may mean routing capital to more environmentally friendly investments.
In manufacturing, sustainability is closely linked to asset performance, resource efficiency and waste management: Is a production line machine consuming more resources than necessary? At what point does an aging assembly line robot turn from asset to liability?
Some level of inefficiency is inevitable in manufacturing. And while no production plant is perfect, improvement is possible by continuously looking to reduce waste. Doing so proactively and at scale can translate into operational excellence and big sustainability gains.
Confronting the issue does not have to require a pricey investment in entirely new assets. Increasingly, manufacturers are pursuing these gains through asset lifecycle management. ALM is a strategy for monitoring and managing manufacturing assets across their entire lifespan and acting on data-driven insights along the way. ALM represents the integration of various processes and technology: routine inspections, a computerized maintenance management system, the wide usage of IoT devices and more.
AI technology has been evolving in parallel with ALM, and some manufacturers are combining the two. From computer vision to machine learning to generative AI, the technology can have an outsized impact on sustainability when coupled with ALM and deployed across industrial workspaces.
Many business leaders are already aware of AI’s benefits for sustainability and are bullish about its adoption: Recent IBM research revealed that 90% of executives believe AI can positively influence their sustainability goals.
However, that ambition doesn’t translate into action: The same research found that fewer than half of organizations are actually leveraging AI for sustainability efforts. Going forward, the most successful manufacturers will be those who act on their AI ambitions and couple the technology with ALM. Here are three ways how.
Computer vision and inspections
Digital technology has made the inspection process more precise and eliminated much of the drudgery. Now, recent advances are compounding these benefits. Computer vision is one of those advances.
Computer vision, a branch of AI, enables machines to interpret objects and is increasingly used in manufacturing. Manufacturers integrate it into their ALM strategies to detect defects and monitor production. Tools like drones, stationary cameras and smartphones equipped with this technology provide real-time monitoring of assets and facilities, enhancing efficiency and identifying issues promptly.
This enables predictive maintenance, another branch of AI, to glean insights from a wide array of sensors and other devices. For example, predictive maintenance leverages both real-time data on an asset’s current condition and historical performance data to provide technicians with advanced warning of potential issues, helping to identify deteriorating health before failure occurs.
The technician can then intervene before it fails and requires a pricey repair bill. This approach allowed IBM and engineering firm Novate Solutions to reduce waste at plants by 15% and improve product quality by 30%. It also enabled IBM and Swedish brewing giant Spendrups Bryggeri to enhance their sprawling maintenance operations.
Generative AI and work order management
Work order management is another part of ALM that has evolved significantly. In most manufacturing settings, piles of paperwork and the clattering of clipboards have been replaced by more effective digital solutions. Still, maintenance managers continue to struggle with assigning proper failure codes and identifying the root cause of issues.
Generative AI is helping solve those issues. Systems infused with generative AI can help with “next action” workflows, creating a failure code recommendation for work orders along with a related predictive confidence score. For example, it may recommend it is 80% confident that an asset failure is related to machine plugging or choking.
To train these AI systems, users only need to label a handful of examples, replacing the tedious task of combing through data sets related to code classifications. This “small data” approach means the technology is more accessible and technicians can troubleshoot problems much more quickly.
Generative AI has applications beyond work orders, too. Manufacturers are using the technology to summarize documents and more quickly design and prototype applications.
AI and energy management
Energy management is also a key part of ALM, ensuring assets are not consuming more energy than they require, driving efficiency down and costs up. Machine learning AI and automation systems can monitor an asset’s energy usage in real time and around the clock, spotting inefficiencies and then proactively recommending changes to reduce usage and costs.
This data-rich approach can be a relief for manufacturers who historically have struggled to quantify sustainability initiatives. Indeed, IBM research reveals that half of business leaders struggle to measure sustainability KPIs.
There is an added benefit of using AI for energy management: It can assist with corporate sustainability rules and regulations. AI for energy management allows manufacturers to carefully track emissions, ensure they meet requirements and better pursue decarbonization.
Looking ahead: agentic AI
Generative AI technology is advancing rapidly, creating even more opportunities for manufacturers to boost sustainability. Evolving agentic workflows level up generative AI from delivering insights to autonomously performing and solving complex tasks set by humans.
AI agents’ ability to interact with external environments enhances productivity and frees up manufacturing staff for more critical and strategic work.
AI is a critical tool throughout manufacturers’ ALM process, from asset planning and deployment to asset operation, optimization and disposal. In addition to the above use cases, manufactures can also leverage AI to create “digital twins” of factories and their production lines, simulating countless scenarios. They can also pair AI with robotics and edge computing — like IBM and Boston Dynamics have — to create roving and artificially-intelligent inspectors.
AI is not a panacea, of course. The technology — like any other — has challenges. Without the right volume and quality of data, AI outputs suffer. And a shortage of AI-skilled professionals means manufacturers do not always have access to the right talent.
These are obstacles to overcome, however, not reasons to eschew AI. Without a doubt, the most successful manufactures this decade will integrate AI widely into their ALM processes.