On one filling line each day, Medivant Healthcare produces 20,000 single dose injectable vials. These vials end up at patient bedsides, on hospital crash carts and in the emergency room, often for patients needing an anesthetic.
The FDA requires that each vial be visually inspected before leaving the plant. Currently, Arizona-based Medivant uses humans to verify that there are no visible particulates or label defects that would render the vials unusable.
But soon, the company’s vial inspection machine will take over that process. “People get tired and bored,” and can miss some defects, while the machines do not, said Medivant CEO Viraj Gandhi.
As part of the machine’s AI training process, Medivant sets standards for what the banned particles look like, to use in the algorithm training, Gandhi added that AI is seeping into many parts of the drug manufacturing process, something the FDA is monitoring. Earlier this year, the agency released a discussion paper, Artificial Intelligence in Drug Manufacturing, requesting comments from stakeholders and the public on how the technology can be best used in the sector.
Integrating AI into drug manufacturing is difficult because the industry is highly regulated, said Thomas Hartman, president and CEO of the International Society for Pharmaceutical Engineering. Any process changes, including the direct or indirect introduction of AI, can impact product quality, requiring the manufacturer to go through a comprehensive change control procedure, which is time and resource intensive.
How drug manufacturing currently uses AI
In addition to quality control, AI can help manufacturers understand how to optimize production processes. AI algorithms can help companies understand how trends and facility temperatures can impact the manufacturing process, including the impact on product quality or yield, said Sue MarchantEVP of product at MasterControl, which sells software for quality management and manufacturing execution.
Some manufacturers use AI to focus on a single manufacturing step prior to commercialization, according to Marchant. During the R&D phase, they run through the manufacturing process changing one step at a time to determine if that improves the quality, results or efficiency.
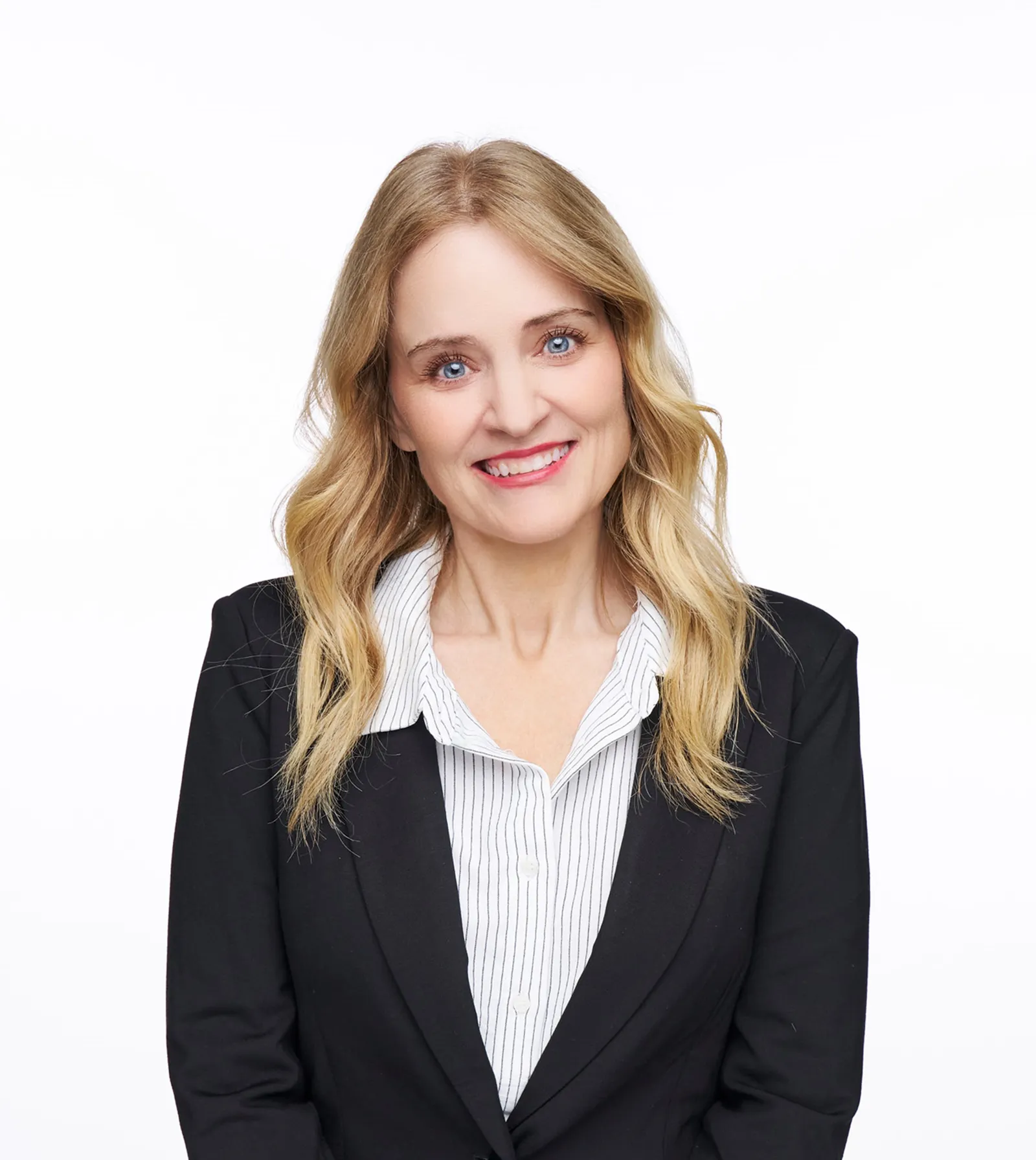
AI can be used to augment a company’s visibility into operations, such as an unplanned deviation on the manufacturing floor. It can also provide contextual data, like sharing other times something similar happened at the facility and what caused it.
“We’re providing [workers] information to potentially guide their actions and assess risk,” Marchant said. “But we’re not yet prescriptively saying we believe you should take this action, so it’s just introducing AI into those processes in a very careful way.”
The technology can be integrated on the quality side, to make it easier for manufacturers to comply with regulations and ensure their practices are safe and secure, by reviewing data and highlighting at-risk areas, Marchant noted.
Challenges implementing AI in drug manufacturing
The biggest roadblock to implementing AI in drug manufacturing is cost, Gandhi said. Medivant spent millions of dollars on its semiautomatic vial inspection, which he says is 10 times more than the cost of human labor for the same job.
“Everyone wants to do this, but you need enough capital,” Gandhi said.
Medivant bought its machine four months ago and is training and validating it with sample vials for four to five months, then will test it with commercial batches. After putting it into production, the company will also perform manual observations for six to nine months, to ensure there are no false negatives in the inspections. Then it will become the manufacturer’s primary inspection method.
Investing in automation and AI is difficult for smaller manufacturing companies like Medivant, which makes generic, low-cost drugs like epinephrine and lidocaine. The company’s products mostly sell for dollars per vial, Gandhi said.
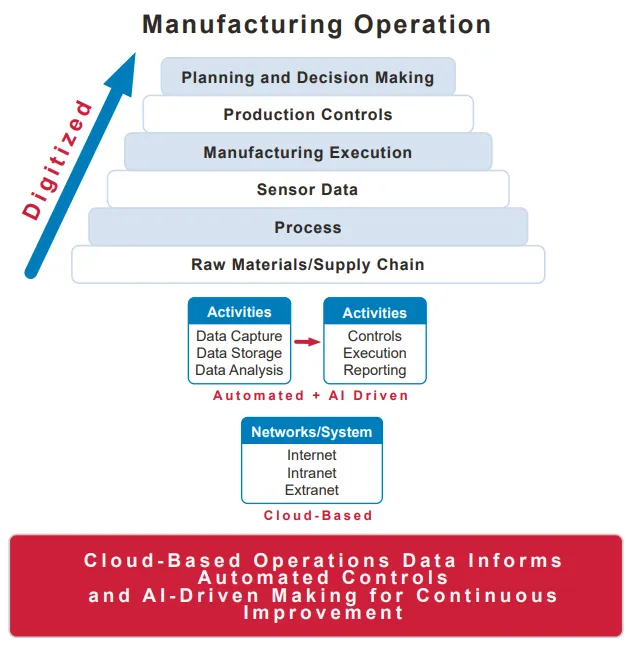
AI tools are, however, more widely adopted by larger manufacturers. “The big ones typically take a risk in new equipment and see how it goes,” Gandhi added.
Another limitation to adopting AI is having the appropriate digitized data available to train the algorithms and run the analyses. This can limit the AI options to larger players, Marchant said, as many smaller manufacturers still use paper logbooks or rely primarily on on-premise data storage, rather than cloud services.
Incorporating AI may require that the “entire manufacturing process is digital, you have outlined every step in your manufacturing process, you are capturing every step digitally, you’re capturing the date and time it occurred, who participated and what the parameters of each step were,” Marchant said. Manufacturers may also need to combine and accurately integrate data from disparate sources.
The FDA’s role
The FDA “has recognized and embraced the potential of advanced manufacturing to bring benefits to patients and consumers,” an agency spokesperson told Manufacturing Dive.
Its Center for Drug Evaluation and Research established the Emerging Technology Program in 2014 to work with stakeholders on supporting advanced manufacturing, including tech such as AI. CDER recognized that regulatory policies and programs may need to evolve to enable timely technology adoption, according to the spokesperson.
“A lot of what we’ve seen [from the FDA] is them trying to get a handle on it,” Marchant said. “There’s not a lot of strong guidance about what companies should do.”
The result, she said, is companies may be hesitant about using AI as they are uncertain what it will mean for them in the future.
“A lot of what we’ve seen [from the FDA] is them trying to get a handle on it. There’s not a lot of strong guidance about what companies should do.”

Sue Marchant
Executive VP of Product, MasterControl
Marchant said her customers are erring on the conservative side, asking in requests for proposals how AI is used in MasterControl software. They are concerned about whether future FDA requirements will interfere with their processes if they adopt AI tools now.
“Everyone’s sort of in a wait and see mode,” Marchant noted. “The FDA definitely wants to encourage innovation, because their message is that they’d like to embrace innovation, and yet what does that really mean? That’s what nobody really knows yet.”
The FDA is interested in incorporating AI in drug manufacturing and highlighted for Manufacturing Dive ways it could potentially improve the process:
• Identify optimal process design and scale-up strategies that might reduce development time and waste
• Gain greater control of product and process quality while reducing human involvement and human error
• Better monitor manufacturing processes and detect changes in performance that trigger preventative maintenance activities and reduce process downtime
• Prevent more process deviations, and in the case of a deviation allow for better root cause identification of the underlying problem
In addition to requesting feedback from stakeholders and the public, in September the FDA cohosted a workshop with the Product Quality Research Institute on the regulatory framework for the use of AI in drug manufacturing.
CDER’s goal, the spokesperson said, is to help patients realize the benefits of using AI in drug manufacturing, while minimizing risks, such as those stemming from the use of AI for unethical outcomes.
The biggest fear, Gandhi said, is that the data can be manipulated. On the inspection side, there is already stringent FDA regulation to validate the accuracy and security of the machine and inspection results.